
While there may be interest or pressure to deploy AI against a company’s most challenging business problem, the ideal project candidates for an initial AI transition are those that consist of fairly simple, repeatable tasks that are typically time and cost-consuming. Be sure there’s ample data to support the project, as well as a way to clearly define metrics for success. When evaluating projects for AI infusion, begin by identifying a narrow, well-defined set of problems that your organization hopes to solve. Knowing where to deploy AIĪs with any digital transformation initiative, knowing where to start is half to battle. For example, in the geospatial analytics and mapping market, computer vision (a specific type of AI) could greatly enhance both the speed and scale in which companies operate. Organizations are increasingly experimenting with AI technology to better understand its return. This may lead some enterprises to wonder, is the transition to AI necessary?ĪI technologies are well-positioned to provide measurable value in the form of speed, accuracy, and/or scale for a wide-variety of public and private sector applications. According to Gartner, between 20, the number of organizations deploying AI grew to just 14 percent.
Topcoder spacenet how to#
This article offers best practices for picking an AI use case, points out barriers to AI success, and advises on how to find the best AI talent.Īrtificial intelligence (AI) technology dominates the headlines, but it’s still not widely used.
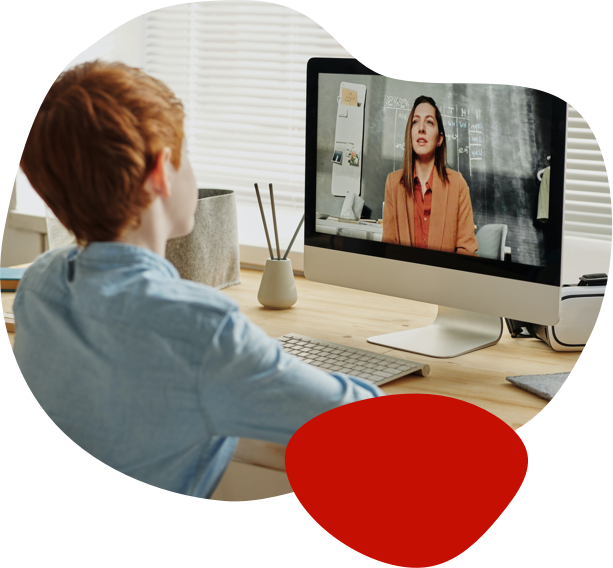
Table 1 shows the breakdown of the type of road by SpaceNet area of interest.AI is poised to deliver measurable value for a variety of public and private sector applications, and is just beginning to make inroads in the enterprise. All roads were digitized from existing SpaceNet data - 30 cm GSD WorldView 3 satellite imagery over Las Vegas, Paris, Shanghai and Khartoum. The new dataset consists of 8,000 km of road centerlines with associated attributes such as road type, surface type, and number of lanes. The SpaceNet Roads dataset was specifically built for the SpaceNet Road Detection and Routing Challenge. For more information and contest rules, visit the SpaceNet Competition page. After the conclusion of the competition, the winning implementations will be open sourced and released on the SpaceNet Challenge GitHub repository. The Challenge, hosted on TopCoder, launched on Tuesday, November 28th and runs through February 2nd. The SpaceNet Road Detection and Routing Challenge: We also created the SpaceNet Roads Dataset, a road network vector layer digitized from SpaceNet imagery to provide accurate ground truth labels. For this challenge, we created a new metric, Average Path Length Similarity, for evaluating the similarity between a ground truth and proposal road network. The Road Detection and Routing Challenge asks competitors to turn satellite imagery into usable road network vectors. The SpaceNet Road Detection and Routing Challenge is designed to assist the development of techniques for generating road networks from satellite imagery.

Machine learning could play a key role in accelerating this process.

Since 2014, over 41,000 contributors have labeled 16 million buildings and manually traced and digitized 13 million km of roadways. In fact, there are large organizations such as the Humanitarian OpenStreetMap Team Missing Maps Project whose entire goal is to map missing areas. Millions of kilometers of the worlds’ roadways remain unmapped. For more information see the SpaceNet Road Detection and Routing Challenge Announcement. Note: SpaceNet is a collaborative effort between CosmiQ Works, Radiant Solutions and NVIDIA.
